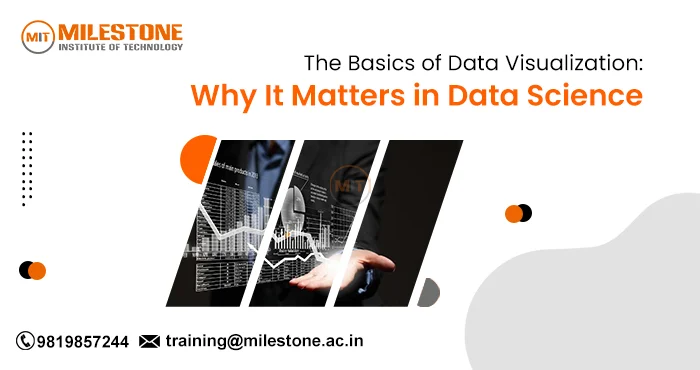
In the present era, we are overwhelmed with data. From social media numbers to the metrics used by businesses, to records kept in health care facilities, as well as environmental figures, data is simply everywhere. But facts and figures in their raw form are just like a disorganized jigsaw, they need to be looked at in a certain perspective. And this is where data visualization comes into play.
When data visualization is discussed in the context of data science, it is often seen as one aspect of the discipline. It’s the link that allows us to combine a quantitative and qualitative understanding of the data at hand. In this article, we will analyze the primary principles of data visualization, attempting to understand its relevance specifically in the context of data science.
So, if you wish to escalate your skills in this area, then a Data Science course in Thane would help in providing you with a systematic approach.
What is Data Visualization?
Data visualization is the simplest way of representing data. This is where charts, graphs, and maps come in as tools to make it absolutely simpler and clearer for one to analyze the particular dataset. It is basically making the numbers and text more appealing to the eye and easy to understand.
For instance, a scatter plot could help in evaluating the changes in data over time while a bar graph could be used in making the comparison on different categories. Large data set can be analyzed through a heat map which explains the overlying pattern in the data. With all these different forms of visualization, the main aim is to clean the data and bring it into use.
So if you want to learn data visualization along with other productive data analytics skills, applying for a top data science course in Thane can be a great move.
- Understanding Data Quickly
Seeing is believing, we are a race that thrives on visuals as we comprehend pictures, illustrations, info-graphics and charts which is about around 60,000 times faster than reading a paper or a book. The good thing about bar graphs and pie charts for example, is that they allow you to see crucial periods or trends in a matter of seconds. And we all know how important data can be considering a lot of decisions are made based on the information one is able to process in a given span of time.
For example, let’s take a data analyst who looks at a lot of sales data for his organization, let’s imagine that there is no data visualization, then for him it would mean that he has to keep looking at rows and columns in a excel sheet trying to find a pattern that tells him the data’s tale. However, they are able to plot a line graph and within seconds they can see figures that tell them the sales trends.
- Uncovering Hidden Insights
Data visualization is not just a process of making data look attractive, it is a process of making information insightful. Sometimes, datasets can have hidden trends or links that are not easily noticeable unless presented in a chart. Efficient visualization is a way of bringing out these relations and allow data scientists to identify patterns that may not be seen at a first glance.
For instance, in customer purchase history, a heatmap will help to understand which goods are more likely to be purchased together. A scatter plot can help in determining if there is correlation between age and the frequency of buying. Such insights can be used to make better business strategies.- Communicating Findings Effectively
- Making Data More Accessible
- Improving Decision-Making
Types of Data Visualizations in Data Science
Data Visualization Types You Must Understand. Now let’s mention some more types of data visualizations. These are frequently applied in data science:- Bar Charts: Fantastic for categories or groups that need to be compared.
- Line Graphs: Best for visualizing changes over history.
- Pie Charts: Ideal for representing parts of a whole.
- Histograms: Show you the shape of a distribution of data.
- Scatter Plots: For exploring the relationships between two sets of data.
- Heat Maps: It’s excellent to show how dense the information is or the correlation between variables.
- Box Plots: Effective to represent the dispersion of the data and also to find values that fall outside the core range.
Best Practices for Data Visualization
Here are a few best practices that will help you present clear and accurate visualizations:- Avoid Complexity: Avoid unnecessary clutter in your charts by keeping your focus on the core message you are trying to convey.
- Use the Appropriate Type of Visual: Different types of data require different types of visuals. For instance, if you’re making comparisons, use bar graphs; for trends, use line graphs; and for fractions or parts of a whole, use pie charts.
- Be Careful with Color: Colors can enhance your message but can also be distracting. Use them strategically to highlight key points.
- Clear your visuals: Ensure your chart’s axes, legends, and titles are comprehensible. This enables your audience to read and interpret your chart without needing you to explain it further.
- Let your visuals do the talking: Good charts do not just present data, they communicate a message. Help your audience understand the visualizations you’re presenting and the key points you’re illustrating.
Conclusion
Data visualization is a key ability for a data scientist. It contributes to, among others, transforming raw data into actionable insights, accelerating analysis, and making the messy and complex into accessible. In the hectic environment of data science, when decisions have to be made quickly, advantage must be attributed to data visualization.
No matter what your level is, a beginner or experience data scientist, sharpening your skill to visualize the data is essential step to achieving the real power of your insights. For those who want to enhance their knowledge of data science, it is recommended to register for a Data Science program in Thane or check out the top data science program in Thane to take your skills to the next level. A properly planned course can give you both the theoretical and practical know how to master these key techniques.